How Can AI Applications Help FinTech Companies
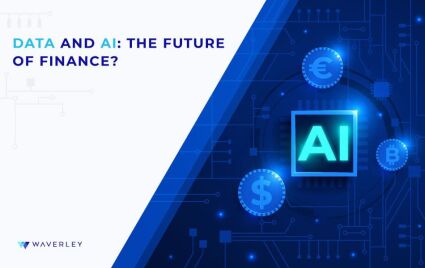
Contents
Artificial Intelligence and Machine Learning are all the rage now in the software development world. More and more organizations are striving to adopt AI in order to follow market trends, keep up with competitors, or attract customers. At the same time, few know how the magic of AI works and that it heavily relies on Data Science and data processing algorithms.
Back in 2016, Forrester stated the collective value of insight-driven businesses would constitute $1.2 trillion by 2020. Following the big data statistics provided by Statista, the big data industry is expected to be worth $77 billion by 2023. In early 2020, Forrester Consulting carried out research “The Business Impact of Data Intelligent Management” which collected answers from around 900 analysts and came to conclude that data-centered businesses are more likely to reach and even exceed their revenue goals by 58%. Finally, in 2020, the total amount of globally created, captured, copied, and consumed data topped 59 trillion gigabytes making a shocking 5,000% increase since 2010.
So what is the role of data for Artificial Intelligence and what’s the effect of AI in fintech market? We will find answers to these questions step by step.
What is Artificial Intelligence?
Generally speaking, Artificial Intelligence is the ability of machines, computers, or robots, to mimic the human way of thinking and behaving. To be more specific, the main human actions AI is meant to reproduce are cognitive functions, such as learning and solving problems, hence the name: Machine Learning and Deep Learning. But in order to perform these actions, Artificial Intelligence needs clear data and the right data patterns to learn from, if we want it to work as intended. AI engineers develop algorithms based on input data and leverage statistical models to predict an output, at the same time updating outputs as new data comes in. This, in turn, is furnished by Data Science.
Many perceive Data Science as a computerized and graphic representation of statistics – with bright and vivid visuals that help easily digest massive pieces of information. Of course, there are great data visualization tools, such as Tableau or Google Charts, for example, that help create very clear and understandable data charts, maps, diagrams, matrices. But you can do much more with Data Science. This is a complex discipline that requires competence in mathematics, information science, computer science, statistics, data analysis, and domain knowledge. The Venn diagram below shows the interrelation of Data Science, Artificial Intelligence and Machine Learning, and other relevant fields.
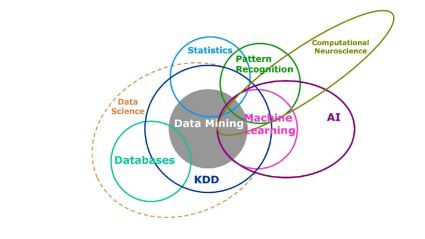
Artificial Intelligence and Data Science
The terms Artificial Intelligence and Data Science are often used interchangeably, and, as can be seen in the diagram, they do overlap in some ways. But they also differ and complement one another in other aspects. For example, Data Science uses structured and unstructured data to find hidden insights and value in these pieces of data. The aim of Artificial Intelligence is to apply this extracted knowledge to a model, or algorithm, and implement this model.
The task of Data Science is to create impacts for businesses. This happens when it helps find a solution to a specific problem. The solution might be a data product, or recommendations for a product, or insights based on collected and analyzed data. These may include, for instance, finding new directions for aligning the company’s strategy, uncovering opportunities, getting more accurate sales forecasts, or improving a product roadmap step-by-step. The value of Artificial Intelligence lies in creating the opportunity to delegate meticulous, dangerous, or even impossible (for humans) tasks to machines that can think like humans: learn based on the received information, analyze it, and come to the right conclusions.
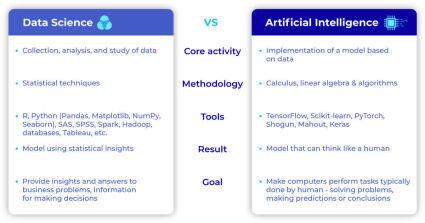
How Artificial Intelligence and Data Science Work
When Data Scientists explain their field of work, they like to draw an analogy to Maslow’s hierarchy of needs. This implies that Data Science is complex and combines various aspects and stages of processing data that rely on each other, including Artificial Intelligence. The hierarchy of needs for Data Science and Artificial Intelligence may also vary from company to company, depending on the business scale, project stage, or even the objectives pursued by the company’s decision makers. Coming to the layers and the roles responsible for these layers in the Data Science hierarchy, or workflow, we can describe six of them:
1) Data Collection
This is the basic need that has to be met for a Data Science project to work. When the problem to be solved is clear, it’s time to collect the right kind of data – the relevant kind. To find the right answer for a specific question, you cannot just use any available dataset. The sources to get data from are numerous: logs, sensors, user-generated content, etc.
2) Data Storage and Management
The next step in a proper Data Science project workflow is making sure the collected data is stored and moved in a reliable manner. Depending on whether you collect Small or Big Data, you will use your own database or additional servers. It’s also important not to collect and store the unnecessary data, noisy or incorrect kinds – so the main task here is to stay focused on the main goal of a Data Science project. Also, structured and unstructured data makes a difference when deciding which database (SQL-based or a NoSQL one) to choose. Building a sustainable data flow, setting up the infrastructure and pipelines in order to make it easy to access and analyze data in the next steps is a part of this need layer as well.
3) Data Munging
After you’ve collected your data and organized the data flow and storage so you could access it, you can start exploring and transforming it. This process includes data exploration, transformation, enrichment, and validation: the data will furnish your request well provided there’s no mishaps or losses. If you happen to discover some gaps at this stage, you can still go back to the beginning and start over.
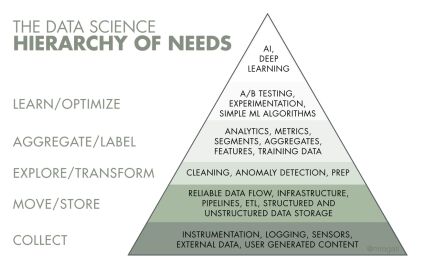
4) BI/Data Analytics
At this stage, once your data is trimmed and polished, you can start analysing. Here, you can classify and label your data sets, define suitable tracking metrics, identify impacting factors, and spot patterns. As a result, you can already outline some key features to be incorporated in your future ML model. You will know what it must help predict or learn, and get down to preparing your training data. At this step, you will also have beautiful charts and data visualizations, but the power of Data Science is not limited to this.
5) Data Optimization
Gradually, your data analytics efforts will grow into a very simple, basic ML algorithm when you do a bit of experimentation and A/B testing. Optimization processes and incremental deployment allow you to get a rough estimate of the possible effects, test the stereotype, and figure out additional features that can affect the final result.
6) Using Your Efforts for AI
Reaching the top of the Data Science needs hierarchy, you can finally build your AI-powered product being certain about your data quality and the ML algorithm accuracy. This hierarchical representation reflects how significant the data preparation process is in achieving the final goals of Data Science or Artificial Intelligence projects.
The roles of Data Science specialists involved in a Data Science or Artificial Intelligence project may vary accordingly, depending on its scale. If you set off with a small team of engineers, one Data Scientist may cover all of the processes, taking care of a small portion of each and taking the responsibility for the data-related part. When your software development project involves a great deal of Data Science processes, this means you need a separate specialist for each process. This way, data collection can be the task of a Software Engineer; data storage, management, and munging is done by a Data Engineer; pure Data Scientists and Analytics typically take care of data analysis and optimization, and the preparation of the basic ML model; the top AI layer is mostly the prerogative of a research scientist, for example, a Deep Learning Scientist, Machine Learning Engineer, or NLP Scientist.
Artificial Intelligence for FinTech
Following the numbers, the combination of AI and Fintech companies has a very solid grounding. For instance, Forbes estimated that by 2025 we will have to process more than 150 zettabytes, that is around 150 trillion gigabytes, of data. At the same time, the financial industry is responsible for generating a considerable portion of this data: a 700% increase between 2016 and 2020. Just take into account the customer data fintech can make use of: deposits and withdrawals at ATMs, point-of-sales purchases, online payments, the collected customer profile data, and so on. What may come as a surprise in this situation, only 29% of the financial industry companies embrace AI&BI in their operations, following the statistics.
How can Artificial Intelligence in Fintech Improve Results?
With this article, we aim to change these sad statistics by explaining the numerous benefits and possible applications of Data Science and Artificial Intelligence in fintech and banking.
Security improvements
Cyber-attackers aren’t stopping inventing new ways to break into computer systems and fraudulent activities are peaking. This means financial organizations remain under the threat of leaking customers’ sensitive data and putting their reputation at risk. In addition, the financial industry is strictly regulated (FATCA, FRTB, Basel III, AML/KYC, MiFID II) which means that organizations must collect and report massive amounts of data in order to meet the rigid standards. New authentication methods, for example, biometric authentication or continuous authentication (tracking the mouse movements and keyboard rhythm), also generate data. Data science can leverage all this data for providing impressive data analytics. On this basis, the ML models and AI for fintech security solutions can be trained to spot suspicious behavior going on in financial systems, draw predictive patterns, and notify security administrators.
Tracking of user behavior and making predictions
There’s a multitude of channels businesses now use for interacting with customers: user-facing mobile applications and web portals, smart devices, social media, vending machines, ATMs, POS machines etc. At the same time, they collect user data, and this is what also makes Artificial Intelligence and Fintech companies good partners. Users’ browsing history, geo-location, interactions, interests, and preferences are invaluable sources of insights. By analyzing this data, companies learn and better understand the needs of their clients, and can adjust marketing and sales efforts accordingly.
Optimization of internal operations
Nowadays, the more cost-efficient your offer is for the customers, the more successful your company may be. But more benefits for customers might mean cutting down on operational needs. If you wish to cut operational costs (without losses), implement automation and data analytics, get some unexpected insights, and train your own predictive models with Data Science and Machine Learning. In the course of operating, you collect and store loads of internal information anyway, so why not leverage it to your benefit?
Getting an advantage over the competition
Quoting Nathan Rothshield, a German banker, businessman and financier, “The one who owns information owns the world.” When dealing with Big Data, we could rephrase it a bit to emphasize it’s important to analyze and use the information you own. Adopting AI solutions and having the insights that your competitors lack, gives you a privilege on the market, makes you stand out in the eyes of your clients as a more reliable and mature partner or service provider.
AI and Machine Learning Applications in FinTech
Now that we’ve considered the benefits of Data Science and Artificial Intelligence for fintech, we can shift our attention to some most common AI use cases in software development for the financial sector.
First of all, there is a commercial component in the operation of any financial organization. Same as other businesses they seek to make profit and achieve this by selling their services and delivering value to the customers. They also have competitors – other fintech companies – so marketing efforts including sales and marketing campaigns, lead generation, communication with customers and finding their pain points are of importance. Let’s take a look at several examples.
Reliable Cyber Security
- With the help of rigidly collected and seasoned data, skillful data analytics efforts, and a well-trained ML model, your computer systems can become more attack-proof. The model tracks user behavior and gradually learns to differentiate between typical and suspicious behavior patterns. As a result, the system prevents further action or notifies users or security managers of possible threats. The cases of suspicious behavior patterns may include unusually high transactions, multiple accounts with similar information appearing within a short period of time, or unusually frequent purchases. An example of a fraud detection mechanism is risk-based user authentication. A fraud-detection engine determines the required authentication level based on the calculated risk profile for each channel request using customer analytics.
- Moreover, like any other computer system, the system of a financial institution also regularly comes under cyber-criminals’ attacks attempting to find an unknown system defect or vulnerability to exploit. The data you collect on your security perimeter will come in handy as well if you apply Data Science and Artificial Intelligence. Physical security items such as surveillance, motion sensors, biometric scanners, and so on are also great sources of useful data to analyze. Make use of it for security purposes with the power of Machine Learning development and Artificial Intelligence tools such as natural language processing or face recognition, for example.
More Efficient Marketing and Sales
- Number one task of marketing efforts is to target the right set of customers. Data Science can help you analyze your existing data and discover patterns, behavior, spending, etc. The clustering technique of data analysis can help segment your audience for a more targeted approach.
- Utilizing user browsing history and social media interaction data in conjunction with Machine Learning, you can spot the best channels to advertise your products and services. AI also helps to automate various processes of interaction with your customers, such as processing customer feedback, and analyzing user journeys. Use the insights you gained to create a more powerful marketing strategy, do advanced key customer network mapping, etc.
- Accurate and well-cleansed historical or test data, in conjunction with predictive analysis models, can help you in forecasting which products or services will spark more interest with your audience, so that you’ll be able to set up dynamic pricing, launch cross-selling projects, and boost your sales strategy.
Personalized Customer Relationships
- Collect relevant data to create accurate user profiles. The multichannel character of today’s business-to-customer interactions allows collecting a ton of personal information about users: their age and gender, family members, civil status, movements, preferences, purchases, day-to-day affairs such as home renovations or starting a business, financial situation, regular income, transaction history, and much more. Use Data Science to analyze user behavior and Machine Learning to adjust your actions accordingly.
- Who wouldn’t like an individual approach? Rely on Machine Learning algorithms to collect and process customer data and personalize your offers much faster and much more efficiently. Making use of customers’ historic data helps to better forecast their further behavior, and lower customer churn rate using the method of logistic regression analysis. As a result, you will know whom you should deliver additional notifications, schedule call-backs, send specific pop-ups, or apply other preventive measures to keep your customers loyal. An interesting example of AI in the Fintech industry is the robo-advisor that offers automated digital investment advice based on the collected customer profile information.
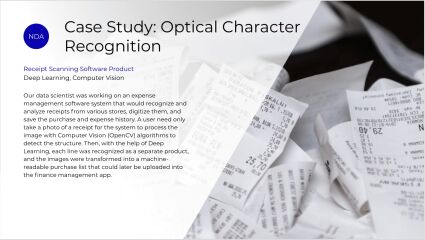
- Lack of timely and humane customer support is often a pain point, so utilizing Data Science can be particularly beneficial in this case. This doesn’t mean you can do without human representatives, though. Automation and accurate data presentation may serve as a great support for your customer support specialist, taking away burdensome workload from them and speeding up their operation while they are on a call with a customer.
- Data Science and ML algorithms can be applied in dealing with large amounts of valuable customer feedback. Take your clients’ criticism and recommendations as your best resource of insights. You can train the system to collect, detect, and analyze meaningful user reports to better understand what your services lack and learn about customer preferences and concerns.
Fintech companies are also risky businesses as they regularly collect large amounts of personal information and deal with enormous sums of money. If you care for the well-being of your customers and the company’s reputation, invest more into a fraud detection mechanism and the protection of critical user data. This task becomes doable with the application of Machine Learning in fintech.
Effective Risk Management
- For investment banks, investment risk modeling is helpful for providing a well-warranted and accurate investment advice. A Machine Learning system can aggregate and process useful data about the objects of investments, such as their state of finances, partnerships, business dynamics and calculate the likelihood of success/failure of the venture. Using these results, bank consultants can be more accurate and give data-backed advice.
- The credit risk modeling approach works with the existing transaction data or banking history (when available) of the person who’s requesting a loan, helping to evaluate their reliability. This enables bank credit consultants to make informed and unbiased decisions, as the process of credit issue is often based on the character of human interaction, with considerable positive or negative emotional impact.
For a financial company, it’s also important that everything runs smoothly inside the business. When it comes to finance and banking, there are many internal factors that may impact the situation: the currency market, the global and local economic situation, political events as well as various regulatory initiatives. When you couple Data analytics with Machine Learning and Artificial Intelligence, dealing with huge amounts of the related data gets much easier with less effort.
Real-time and predictive analytics
- Real-time analytics is useful when financial companies want to analyse their running internal processes, by taking a look at their current, most recently collected data. This way, they can use fresh external financial data such as currency exchange rates, stock market fluctuations information about recent investments, etc. to draw timely insights and make data-driven decisions.
- With predictive analytics, you can forecast potential risks or prospects. Your historic data as well as current internal and external data will come in handy in this case. This type of analysis is helpful for business leaders in strategic planning, process refinement, finding new more efficient approaches or parting with old-fashioned practices.
Conclusion
Taking into account the steep digitalization of all the daily interactions between individuals and businesses, there’s no doubt that the amount of generated data will only continue to grow, no need to ask the statisticians on this one. And there’s nothing else left to do for companies but leverage this data for the benefit of businesses and customers. In this article, we’ve covered just the most common cases for Data Science, Machine Learning, and Artificial Intelligence in Fintech – there is much more still to be discovered and invented.
Waverley Software has been a reliable software development partner with strong expertise in Data Science and Artificial Intelligence for almost three decades now. Our software engineers are well-versed in a range of AI and ML tools, including databases, frameworks, and libraries. We can offer our skills and knowledge to Fintech startups as well as enterprises and established businesses: we always enjoy building new and creative projects. Explore our AI development service page to learn more about our Data Science and AI expertise, projects, and offers.