Integrating AI for Improved Business Intelligence
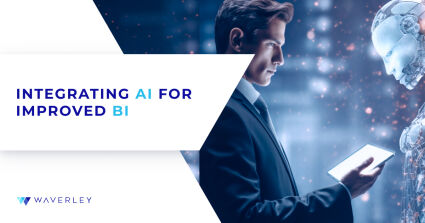
Contents
Modern tech can take your business to the next level. By leveraging artificial intelligence (AI) in combination with business intelligence (BI) you can uncover powerful business insights and enhance your business strategy. Whether used for identifying trends, simplifying complex tasks, or streamlining work processes, AI could transform the capabilities of BI to make it more accurate, effective, and innovative.
At Waverley Software we understand and emphasize the importance of BI in advancing business growth. Integration of AI and business intelligence has the ability to transform the way your business operates. In this post we’ll focus on the power of BI and AI, the difference between AI and BI, what unites them, and most importantly how AI can enhance BI practices.
Understanding the Integration
The evolution of artificial intelligence has emerged as a game-changer in the realm of business intelligence. How? What exactly did AI improve in BI practices and solutions? How to use AI for business intelligence? First, let’s have a look at AI and BI separately. What is AI and BI? The terms Artificial Intelligence (AI) and Business Intelligence (BI) are not interchangeable.
According to E. Foley and M. G. Guillemette BI refers to the technologies, processes, and tools that organizations use to collect, analyze, and present business data to support decision-making processes. There are many benefits of the business intelligence, but the primary goal is to transform raw data into meaningful insights, helping businesses make informed and strategic decisions.
Key Components of BI
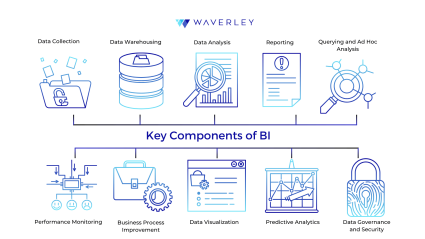
Here are the key components of BI in detail:
- Data Collection: Gathering data from various sources such as internal databases, external databases, spreadsheets, and more. Extracting, transforming, and loading (ETL) processes to ensure data consistency and integrity.
- Data Warehousing: Storing and organizing data in a centralized repository (data warehouse) for easier access and analysis and creating data models that facilitate efficient querying and reporting.
- Data Analysis: Employing analytical tools and techniques to explore and analyze data. After generating reports, dashboards, and visualizations to communicate insights effectively.
- Reporting: Creating and distributing reports highlighting key performance indicators (KPIs) and trends. Enabling stakeholders to monitor business performance and make data-driven decisions.
- Querying and Ad Hoc Analysis: Allowing users to interact with data through queries and ad hoc analysis to gain specific insights. This component also allows supporting self-service BI, where non-technical users can access and analyze data independently.
- Performance Monitoring: Tracking and monitoring key metrics and performance indicators in real-time and setting up alerts for anomalies or deviations from established benchmarks.
- Business Process Improvement: Identifying areas for improvement based on data insights, as well as supporting strategic planning and forecasting through data-driven decision-making.
- Data Visualization: Representing complex data sets through charts, graphs, and other visualizations to aid understanding. Additionally, enhancing the communication of insights to various stakeholders.
- Predictive Analytics: Using statistical algorithms and machine learning to forecast future trends and outcomes and enhance decision-making by providing insights into potential future scenarios.
- Data Governance and Security: Establishing policies and procedures for ensuring data quality, accuracy, and security and complying with regulations and standards to protect sensitive information.
Overall, BI tools and processes empower users at all levels of an organization to access and interpret data, fostering better decision-making and overall business success.
Key Components of AI
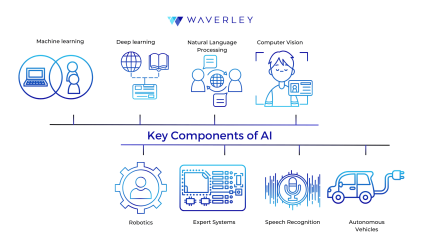
Regarding AI, during the 6th International Conference on Computer and Technology Applications AI was defined as a simulation of human intelligence in machines that are programmed to think and learn like humans. The goal of AI is to enable machines to perform tasks that typically require human intelligence, such as visual perception, speech recognition, decision-making, and language translation.
Let’s have a look at AI’s main branches in more detail:
- Machine Learning (ML): ML is a subset of AI that involves the development of algorithms and statistical models that allow computers to perform tasks without explicit programming. It relies on data to train models and improve their performance over time.
- Deep Learning: Deep Learning is a specific subset of machine learning that uses artificial neural networks to simulate human-like decision-making. It excels in tasks such as image and speech recognition.
- Natural Language Processing (NLP): NLP enables machines to understand, interpret, and generate human language. It is used in applications like chatbots, language translation, and sentiment analysis.
- Computer Vision: Computer Vision involves teaching machines to interpret and make decisions based on visual data. Applications include image and video analysis, facial recognition, and object detection.
- Robotics: AI is applied to robotics to create intelligent machines capable of performing tasks autonomously. Robotic process automation (RPA) involves automating repetitive tasks using AI-driven robots.
- Expert Systems: Expert systems mimic the decision-making ability of a human expert in a particular domain. They use rules and knowledge bases to make decisions and solve problems.
- Speech Recognition: AI is used to convert spoken language into written text, enabling voice-activated systems and voice assistants.
- Autonomous Vehicles: AI is crucial for developing self-driving cars and other autonomous vehicles, allowing them to perceive their surroundings and make decisions in real time.
- Cognitive Computing: Cognitive Computing involves creating systems that can understand, reason, and learn like human cognition. It aims to simulate human thought processes in solving complex problems.
AI and BI are two powerful technologies that have the potential to transform how businesses operate and make decisions. While BI focuses on analyzing historical data to gain insights and support decision-making, AI enables machines to learn from data, make predictions, and automate tasks.
Using AI in Business Intelligence
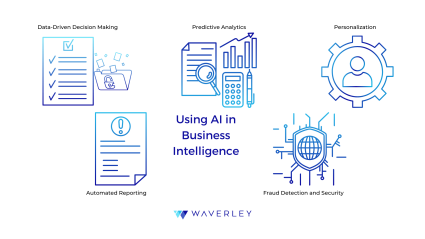
- Data-Driven Decision Making:
BI facilitates the collection, organization, and analysis of diverse data sources. When combined with AI, it empowers businesses with deeper insights, fostering data-driven decision-making across all levels. By leveraging BI and AI, companies can effectively mine valuable information from their data, identify patterns, and predict trends. This synergy enables them to make informed choices, optimize processes, and gain a competitive edge in the market. The integration of BI and AI is a powerful tool for modern businesses, driving efficiency and strategic decision-making through the transformative capabilities of data-driven insights. - Predictive Analytics:
With the help of AI-powered algorithms, businesses can delve into historical data to forecast future trends and outcomes effectively. These predictive analytics play a crucial role in anticipating demand fluctuations, identifying potential risks, and optimizing operational processes. By harnessing AI’s capabilities, companies gain the ability to make proactive decisions, mitigate risks before they escalate, and capitalize on emerging opportunities.Predictive analytics empowers businesses to stay ahead of the competition by aligning their strategies with anticipated market shifts. Updated Technical Training Programs also play a vital role in empowering employees. This data-driven approach not only enhances operational efficiency but also fosters a customer-centric approach, enabling businesses to cater to evolving consumer preferences and needs.
Gartner analytics ascendancy model explicitly shows how data analytics has evolved.
- Data-Driven Decision Making:
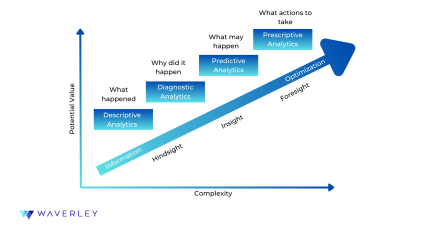
- Personalization:
AI analyzes customer data to understand preferences and behaviors, enabling businesses to tailor products and services. BI measures the effectiveness of these efforts, ensuring precise targeting and enhancing customer satisfaction. With personalized offerings, businesses can strengthen customer relationships, drive loyalty, and boost revenue.By combining AI’s insights with BI’s evaluation, companies refine their strategies to meet individual needs, positioning themselves for success in competitive markets and framing good Technical Program Management Training for employees.
- Automated Reporting:
Integrating AI with BI streamlines report and dashboard generation, freeing up employees to focus on insightful analysis rather than manual reporting tasks. Time saved by automation enhances productivity and decision-making efficiency. With AI’s ability to process vast datasets rapidly, businesses receive real-time updates, enabling timely responses to emerging trends.Automated reporting not only expedites data-driven decision-making but also ensures accurate and consistent reporting across the organization, fostering a cohesive understanding of performance metrics and facilitating strategic planning for the future.
- Fraud Detection and Security:
AI algorithms excel at spotting anomalies and potential fraud within extensive datasets. Integrating AI with BI empowers businesses to fortify their security measures by swiftly identifying suspicious activities and potential threats. By leveraging AI’s proactive detection capabilities and BI’s comprehensive data analysis, companies can strengthen their defense against cyber threats, safeguard sensitive information, and protect their reputation.This powerful union ensures a robust security framework, instilling confidence in customers and stakeholders alike, and mitigating financial and reputational risks associated with fraud.
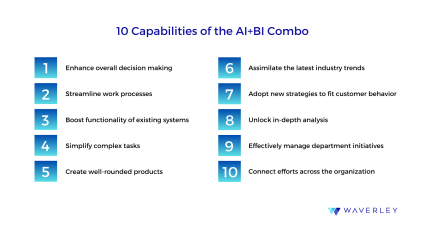
Reasons for Collaboration
It’s not exactly easy to take advantage of the collected data. Here lies the main reason for using AI in business analytics. Integration of AI and business intelligence can be the game changer here.
According to BARC Survey ‘The Future of BI & Analytics’ companies and organizations can expect to gain certain benefits from utilizing generative AI in business intelligence.
AI is capable of performing tasks close to human intelligence abilities like visual and auditory perception, NLP, pattern recognition, and decision-making, but comprehensively faster and at a larger scale. Therefore, AI-enabled business intelligence tools can analyze large amounts of data in real-time, generate insights, structure data, anticipate trends, or make recommendations.
AI and BI collaboration come in different forms and shapes. Let’s have a closer look at this union.
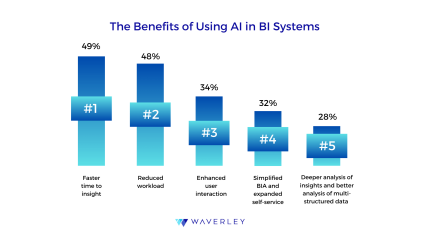
- Augmented Analytics for Simplified Complexity
Augmented analytics is known for democratizing data and reducing the time needed to build ML models. It enhances the use of data, automating routine manual tasks across the data analytics lifecycle, and shortening the time between the moment you collect the data and act on it. - Cognitive Search for Efficient Data Discovery
Cognitive search, or AI search is a type of search technology that uses AI to understand and anticipate the user’s needs and intentions. It can deliver relevant and contextual information to the user by analyzing the patterns and relationships that exist within the data. The traditional search has been shown to have certain challenges such as unstructured data, poor search queries, and limited human bandwidth.AI search is a progressive improvement and a radical shift in how we find information. The cognitive search comes with these benefits:
- enhances query relevance, thereby boosting productivity
- provides precise results by understanding natural language and context
- personalizes outcomes through machine learning, fine-tuning its accuracy and speed over time
It is predicted that generative AI search, exemplified by Retrieval Augmented Generation (RAG), is the next step of cognitive search. It is a concept that merges the intelligence of AI search tools with the creativeness of generative models (like ChatGPT). This powerful combination promises a seamless and intuitive search experience that answers queries and provides precise references.
- Automated Report Generation
Automated reporting is about bringing users relevant useful information in a timely way, without the users having to seek out the information for themselves. It tells you what has happened and how different areas of a business are performing. Automated reports can be generated at fixed intervals. They may also be triggered by certain events, like a shipping backlog that has now increased to a critical level that must be resolved. But how can BI and automated reporting work together?A business intelligence application with built-in automated reporting can bring you the advantages of both approaches. By using the BI application as a single data repository, everybody can analyze the same versions of the numbers and work from a single source of truth to get information and insights. Simple connection of multiple data sources and easy creation of reports and dashboards let your users get what they need and get on with their jobs, with little or no help from IT.
- Adaptive Data Governance
AI tools can help organizations adapt their data governance policies dynamically. Which means they are based on changing business requirements, regulatory standards, and risk factors. By analyzing trends, patterns, and emerging threats in data usage, AI-driven analytics can inform adaptive policy management strategies, ensuring that data governance remains agile and responsive to evolving needs.More importantly, AI-powered techniques such as machine learning and natural language processing can enhance data privacy and security by identifying and mitigating risks associated with sensitive data exposure or breaches.
- Automated Anomaly Resolution
Since AI algorithms can analyze vast amounts of data in real-time it allows detecting anomalies more accurately than traditional methods. With AI-powered anomaly detection techniques, BI systems can identify deviations from expected patterns or behaviors with greater precision, reducing false positives and improving the accuracy of anomaly detection. - AI Boosts BI functionality
The real potential of Business Intelligence can be gauged by breaking down a large volume of data into granular insights. It enables enterprises to comprehend smaller aspects of the big picture. AI boosts the capacity and functionality of BI applications too. However, the real impact lies in real-time insight. Actually, BI’s mainstream process is to process and visualize data. However, BI can not generate this data result and predict trends in real time. AI with the combination of the latest technologies like ML infuses the capacity of generating real-time insights and trends. Boosting BI functionality improves the value to the organization.
How AI Can Transform Business Intelligence
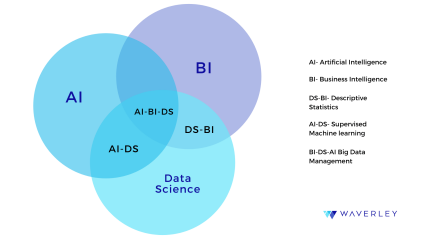
AI in business intelligence is making it more accessible to a wider range of users. AI-powered BI tools can be used by business users who do not have any coding or technical expertise. This is because AI-powered BI tools can understand and respond to natural language queries. Let’s look at examples and specific ways in which AI is transforming BI.
Use Case | Description |
---|---|
Automated data preparation and cleansing | AI can be used to automate the process of preparing and cleansing data for BI analysis. This can save businesses a significant amount of time and effort, and help to ensure that the data is accurate and reliable. |
Natural language processing (NLP) for data exploration | AI-powered NLP can be used to enable business users to explore data using natural language queries. This makes BI more accessible to a wider range of users and can help uncover insights that would be difficult or impossible to find using traditional methods. |
Machine learning (ML) for predictive analytics | AI-powered machine learning services can be used to build predictive models that can be used to forecast future trends and outcomes. This information can be used by businesses to make better decisions about everything from product development to marketing strategy. |
AI-powered BI dashboard | AI can be used to create BI dashboards that are more personalized and interactive. AI can also be used to provide users with real-time insights and recommendations. |
Cybersecurity | AI is an indispensable ally in preventing and avoiding network security threats. AI systems can recognize cyberattacks and cybersecurity threats by monitoring data input patterns. After detecting a threat, it can backtrack through your data to find the source and help prevent future threats. AI is an extra set of diligent, constantly seeking eyes that can significantly bolster your infrastructure. |
CRM | AI is also changing CRM systems. Typically, CRM software requires significant human intervention to remain current and accurate. However, today’s best CRM software uses AI to transform into self-updating, auto-correcting systems that do much of the background work of managing customer relationships. |
Another way of AI changing your business is by using it as a digital personal assistant. To be specific, AI can transform internal business operations through AI chatbots or ChatGPT integrations that act as personal assistants, helping to manage emails, maintain calendars, and provide recommendations for streamlining processes. Additionally, chatbots can help you grow your business by handling customer inquiries online.
By offloading various tasks to chatbots, you improve customer service while gaining extra time to focus on strategies to grow your business.
AI and Workers
Since AI sets new trends for the industries, the question is how will it affect the workplaces? According to the Business News Daily, these are the trends we might see in the future.
- AI may affect analyst jobs
- AI may create more jobs
- AI may require a shift to more specialized skills
- AI-created jobs may disappear in the future
AI+BI: Real-World Use Cases
Looking at real-life examples we are faced with the question of how to integrate AI into your business process. Let’s a have better look at examples of AI in business intelligence applications or platforms. A number of businesses have successfully leveraged the integration of AI and business intelligence.
Application | Description |
---|---|
SAP – Transforming Databases Into Useful Intel | The major AI benefits in business intelligence can be seen in HANA- SAP’s cloud platform that enables you to manage databases of collected information. The solution ingests and replicates the data from a variety of sources such as apps, databases, and more. Users can access the platform via both on-premise servers and the cloud. HANA is potential enough to include information from financial transactions, equipment, sensors, and desktops. Suppose your company staff uses a smartphone or tablet to record purchases; then, they can leverage HANA to identify trends and irregularities. |
DOMO – AI for Business Dashboards | Domo, being a business management software company, it has raised more than $500 million in funding and has developed a dashboard that collects data to aid companies in making actionable decisions. This cloud-based dashboard can scale the size of the company easily. So, both small and large enterprises can leverage the system easily. There are 400+ native software connectors that enable Domo to collect data from a third-party application that can be leveraged to table insight and context. |
Avanade – AI for Business Insights | Avanade is a program jointly initiated by Accenture and Microsoft that is based on Cortana Intelligence Suite and many other solutions for predictive analytics and uncovering data-based insights. The platform helps companies to gain more perspective and insight into the business. You can learn about consumer behavior through analytics. |
Waverley Software: Success Stories
Here at Waverley Software, we have an experienced team providing BI consulting services. They work closely with AI engineers to reach maximum results by integrating AI and business intelligence.
Toyota InfoTechnology Center | Componentality |
---|---|
Waverley started working with Toyota’s R&D team back in 2013 with the initial project of early adoption of the first Apple CarPlay and Android Auto SDK with the Toyota Platform, a Linux-based car Computer. It was a challenging and collaborative effort that led to a successful Demo at Toyota’s HQ in Japan. The business success of the project was overseen by our BI consulting team. | Componentality is an automotive Research and Development company, that creates passenger-oriented devices for public transportation, provides entertainment and connectivity for cars and road infrastructure, and develops unique technical solutions for special purposes in the automotive field. The company reached out to Waverley for help with BI services and the firmware part. As a result of the project, our team was able to create applications and components of the OS for the car computer and help to integrate the system with mobile phones. |
Challenges and Solutions
With all the opportunities offered by AI in business analytics, a number of challenges may arise.
- Poor Data Quality. Having outdated or poor-quality data may lead to a process of optimization being inefficient.
Solutions could be found in these processes: data profiling, data cleansing, data standardization, automated data quality checks, and data validation. - Lack of Technical Skills. Using AI in business intelligence requires a high level of technical expertise, that can prevent many companies from implementing BI and AI within their organizations.
There is no solution other than training and skill development, collaboration with external partners, or creating cross-functional teams. - High Implementation Costs. As new emerging technologies the cost of integrating AI into BI (technology, training, expertise) could be significant.
A part of the solution is to focus on your Return on Investment, because if implemented wisely, those modern solutions can bring a lot of value to your organization in the long run and make you more competitive. Also, when integrating BI and AI, we usually recommend our clients to start small.. Instead of trying to implement AI and BI solutions across the entire organization at once, start with a small-scale pilot project or proof of concept. To reduce costs, try resorting to outsourcing companies or external consultants.
Tips for Implementation
As we stated above, integrating AI into BI can enhance the capabilities of BI systems by adding predictive and prescriptive analytics, automated insights generation, and more advanced data processing techniques.
To reach maximum results from enhancing your BI services and ease the implementation process it is recommended to follow these steps.
- Define Clear Objectives
Clearly define the objectives and goals you want to achieve by integrating AI into your BI system. Determine specific business problems you want to solve or the insights you want to uncover using AI-powered analytics. - Evaluate the Quality of Data
Evaluate the quality, completeness, and relevance of your data. AI algorithms rely on large volumes of high-quality data, so you need to ensure that your data is clean, well-structured, and properly labeled for training AI models. - Choose the Right AI Techniques
Select AI techniques and algorithms that are well-suited to your business objectives and data characteristics. Common AI techniques used in BI include machine learning for predictive analytics, natural language processing for text analysis, and computer vision for image analysis. - Identify Use Cases
Identify specific use cases where AI can add value to your BI system. This could include predicting customer behavior, forecasting sales trends, optimizing pricing strategies, or automating data processing tasks. - Integrate AI Models or Create Your Own
Integrate AI models seamlessly into your existing BI infrastructure. This may involve leveraging APIs or SDKs provided by AI platforms or customizing your BI tools to support AI capabilities. - Provide User-Friendly Interfaces
Design user-friendly interfaces that make it easy for business users to interact with AI-powered features within the BI system. This could include dashboards, reports, and visualization tools that present AI-driven insights in a clear and understandable manner. - Ensure Transparency and Explainability
Ensure that AI-driven insights are transparent and explainable to users. Provide explanations or visualizations that help users understand how AI models arrived at their conclusions and recommendations. - Stay Agile and Adaptive
AI technology is rapidly evolving, so stay agile and adaptive in your approach to integrating AI into BI. Keep abreast of the latest advancements in AI and BI technology and be prepared to adapt your strategies accordingly.
Explore Emerging Trends in AI and BI Collaboration
Over the past decade, business intelligence has changed considerably. 2023 was a particularly significant year for the business intelligence industry. In the comping years BI tools and strategies will become increasingly customized. Businesses of all sizes are no longer asking if they need increased access to business intelligence analytics, but what is the best BI solution for their specific needs?
But first, let’s have a look at BI statistics in numbers.
- As per Markets and Markets, the business intelligence market will be worth $33.3 billion by 2025, growing at an annual CAGR Of 7.6%
- The global mobile business intelligence market was valued at $8.8 billion in 2020 and is expected to reach $29.6 billion by 2027 at a CAGR of 18.9%.
- The worldwide business intelligence software market is projected to reach $17.6 billion by 2024 when run together with analytics tools, as per Statista.
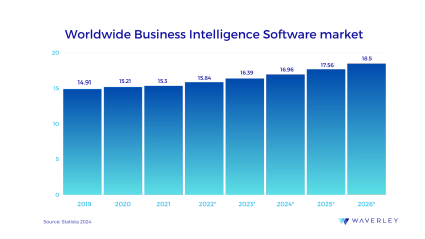
Experts outlined a few emerging business intelligence and analytics trends in 2024.
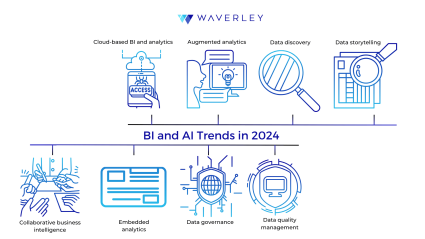
BI and AI Trend | Description |
---|---|
Cloud-Based BI and Analytics | Cloud-based BI is a key business intelligence future trend. Most businesses’ BI elements, such as data sources, models, analytics, computing power, and data storage, have already moved on to the cloud where its adoption has led to better collaboration and productivity amongst their workforce. Businesses must harness the power of cloud analytics to garner actionable, timely insights from the data. |
Augmented Analytics | According to Markets and Markets, the global augmented analytics market size is expected to reach USD 23.27 billion by 2024. It is one of the critical components in the future of data that leverages the power of machine automation and AI by transforming the way data is generated and sharing valuable insights. The evolution of natural language query capabilities has driven up the adoption of BI among business users while delivering analytics has become a vital functionality for BI vendors. Analytics platforms have even started embedding Artificial Intelligence (AI) and Machine Learning(ML) capabilities in business workflow for better insight delivery. |
Data Discovery | Data discovery is among the top business intelligence trends in the importance hierarchy. Studies have shown that the empowerment of businesses is possible with proper data discovery techniques and visualization as it produces relevant insights for the long-term decision-making process. Data discovery has increased its impact over the years by detecting patterns through visual analysis, guided advanced analytics, and accurate data preparation. |
Data Storytelling | Data storytelling has become pivotal as it can add context and a better understanding of statistics by providing a business with the picture needed to put their insight into action. Dashboard figures alone do not make sense anymore, as they need to be interpreted or contextualized. This is where storytelling comes into the picture around data. This trend will change progressively in how businesses can use data to engage better, acquaint themselves with the unknown, and try new ideas. |
Collaborative Business Intelligence | Collaborative information and decision making is the key focus of new BI solutions. It combines collaboration tools with online BI tools, including social media and other 2.0 technologies like Ajax and JavaScript. These BI tools can make sharing easier as automated reports can be scheduled at specific times and to people with a flexible level of interactivity. |
Embedded Analytics | As per Allied Market Research, the embedded analytics market is projected to reach about $77.52 billion by 2026, with a CAGR of 13.6% from 2017. Embedded dashboards have enormous potential as they provide better value within their application. Businesses can create a sales report or send multiple dashboards to clients with the help of embedded analytics which is becoming a standard norm in business operations. |
Data Governance | Data Governance has become an indispensable part of business excellence as stringent security and data privacy measures are brought into focus. It has now become essential for any business that wishes to get significant returns from business intelligence investment. With the passage of the General Data Protection and Regulation (GDPR), businesses must implement appropriate data governance programs and remain compliant with relevant laws. |
Data Quality Management (DQM) | Data Quality Management is a combination of technology, process, people, and culture that delivers accurate and valuable data with the help of a wide range of metrics used for analysis. It improves the data governance framework of an organization by ensuring standards are maintained, and stakeholders have a clear picture of day-to-day operations. As a result, business leaders can make the right decision to move their businesses forward. |
Conclusion
AI-powered data collaboration platforms represent a significant investment for businesses. Especially if the businesses want to stay competitive in the near future. By facilitating efficient data collaboration, they enable businesses to harness the potential of their data, develop insights that lead to better decision-making, and achieve operational efficiencies. As businesses would need to process and present data into visual findings, software that can make exact trend predictions in real time would be of major significance. AI can show its real potential there and enable businesses to seek a breakthrough in the market.
If you want to be sure how to use AI and power BI and how to implement it within your business our specialists at Waverley Software will guide you through.
FAQ
How does AI complement traditional BI practices?
AI complements BI practices by improving data analysis, predictive capabilities, and decision-making processes. This is how AI enhances BI practices: integration with IoT and big data, error detection, and risk management, NLP technologies, predictive analytics and modeling.
What is the significance of integrating AI with Business Intelligence?
There are multiple reasons why the integration of AI and business intelligence is significant. One of them is operational efficiency. By integrating AI-driven automation capabilities with BI platforms, businesses can streamline workflows, reduce costs, and improve efficiency. Another vital reason is predictive analytics. AI-powered algorithms can analyze large volumes of data more efficiently and effectively than traditional BI tools alone. We can also add personalized experiences to customers and enhance data analysis.
How is AI used in business analysis?
The most common ways to use AI in business intelligence are data analysis and visualization, predictive analytics, NLP technologies, pattern recognition, automation of repetitive tasks, customer segmentation, market research, and risk management.
How has AI changed the analytics?
AI has modernized and revolutionized analytics by introducing advanced capabilities, automation, and scalability. This allows businesses to derive deeper insights, make more accurate predictions, and gain a competitive edge in today’s data-driven world.
What role does AI play in making BI more accessible to non-technical users?
AI plays a great role in making BI way more accessible to non-technical users. How? By simplifying data analysis, automating tasks, and providing intuitive interfaces. For example, automated recommendations, smart alerts and notifications, personalized user experiences, or data visualization tools. These functionalities are now easily available for non-technical users.
Can AI-powered BI systems handle real-time analytics?
AI-powered BI systems are well-equipped to handle real-time analytics by leveraging AI algorithms, machine learning techniques, and streaming data processing capabilities.
Transform your BI with AI innovations.