AI Transformation Guide: Implementing Success Strategies
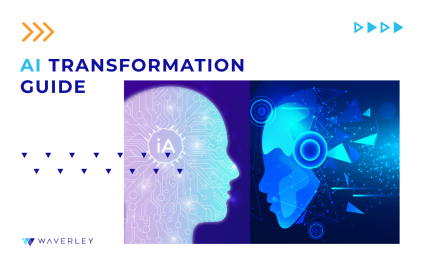
Contents
Embracing the power and opportunities of artificial intelligence (AI) has become increasingly essential for businesses looking to gain a competitive edge, drive innovation, and unlock new growth directions. Having said that, starting an AI transformation journey might be daunting, especially for organizations unfamiliar with AI technologies. Where to start?
We have prepared for you a complete AI transformation guide where you can find all the answers you need, including what AI transformation and its key components are. We will guide you through the steps of digital transformation and discuss the emerging trends for the following year.
What is AI Transformation?
Simply put, AI transformation is the next phase of digital transformation, that aims to integrate AI solutions into all areas of a business. What for? To improve the company’s value proposition to customers and transform day-to-day operations. Moreover, when we look at data and statistics, it’s clear that AI adoption touches virtually all aspects of the modern enterprise and can be very beneficial in both commercial and operational aspects.
For example, according to a 2023 Gartner Survey, 79% of corporate strategists define AI, data analytics, and automation as the key to success over the next two years. Meanwhile, Moody’s survey of 550 compliance and risk leaders and professionals from around the globe revealed that 8 out of 10 of them expect the adoption of AI within the next 5 years. And PWC predicts GenAI will have a substantial impact on businesses at all levels. No wonder automated emails and chatbots like chatGPT are two of the most common uses of AI in everyday business communications.
Also, highlighting the role of AI in digital transformation, Analytics Insight reports that 80% of retail executives expect their businesses to adopt AI automation by 2025. Generative AI can improve a highly skilled worker’s performance by as much as 40% compared with workers who don’t use it. The Future of Jobs Report predicts that by 2025, as many as 97 million people will be working in AI.
Finally, AI is projected to achieve a CAGR of 52% by 2025, indicating its rapid adoption by worldwide businesses. The global machine learning market size was valued at $19.20 billion in 2022 and it is projected to reach $225.91 billion by 2030 with a CAGR of 36.2%.
As we can see, there is no slowing down in the pace of AI and digital transformation as it helps businesses deliver highly personalized digital customer experiences, enable the emergence of new business trajectories, and incite the companies’ race for innovations. Thus, businesses and companies need to choose the right AI strategies for business transformation, while the integration of AI is permeating all spheres of life and being put into action across diverse industries, including but not limited to national security, healthcare, logistics, and education.
In our earlier article, we discussed how to integrate AI into your business. This time, we will guide you through the role of AI in digital transformation and the key components of the AI transformation process.
Key Components of AI Transformation
AI transformation of a business is a complex process that involves different underlying tools and technologies. In this section, we briefly outline the key components of AI transformation that shape the way AI technologies will be implemented in the organization.
1. Machine Learning Algorithms
Machine learning algorithms are collections of very complex calculations done using certain data sets to train the computer system to come to certain conclusions, based on that data. For example, with ML, computer systems can learn to identify specific objects and classify them, spot patterns and make generalizations, extract meaning from natural language, and more. Thus, with machine learning development, computers are trained to complete different cognitive tasks that could only be done by humans some time ago – such as natural language processing (NLP), Computer Vision, prediction, or decision-making.
There’s also a more advanced version of ML – Deep Learning, which relies on neural networks and can complete highly demanding tasks. These algorithms serve as the engine powering intelligent applications and systems, enabling organizations to extract insights, automate processes, and enhance decision-making.
2. Data Analytics Infrastructure
Making sure that the data infrastructure is ready can significantly impact the effectiveness and success of your AI implementation. For AI systems to deliver accurate results, they need access to high-quality, clean, and relevant data. The first step is to collect relevant data from different sources within your organization, such as customer databases, transaction records, social media analytics, and operational data.
Once the data is collected, it needs to be cleaned. Cleaning data involves removing duplicates, correcting errors, dealing with missing values, and ensuring data consistency. The cleaned data should provide a complete, accurate, and consistent view of the business aspect you need to boost using AI, for example, operations, customer interactions, market trends, etc. With your data cleaned and ready, it’s time to focus on the infrastructure — the hardware and software required to run AI systems.
3. Automation Technologies
Automation technologies play a pivotal role in streamlining workflows and augmenting human capabilities, amplifying productivity and efficiency. For example, autonomous IT operations can enable self-healing systems, autonomous infrastructure management, and predictive capacity planning, reducing the need for human intervention and letting IT teams focus on higher-value tasks.
4. Cloud Computing Infrastructure
Cloud computing infrastructure serves as the backbone, offering scalable and cost-effective resources to support AI workloads. The relationship between cloud computing and AI is highly beneficial for organizations looking to digitally transform. There are some ways in which these two technologies work together:
- Data storage and access
AI requires access to large datasets to train and improve its models. Cloud computing provides the scalable storage needed to house this data and facilitate global access. - Computing power
Training AI models can be computationally intensive. Cloud service providers offer scalable, high-performance computing power to accelerate the AI training process, enabling organizations to complete tasks faster and at a lower cost. - Agile implementation
Once AI models are trained, they must be implemented in production environments. Cloud computing facilitates this implementation and helps organizations scale and manage their AI applications efficiently.
Together, these components form the bedrock of AI transformation for software companies, empowering them to innovate, differentiate, and thrive in an increasingly AI-driven landscape.
Other important factors that ensure successful AI-driven digital transformation are:
- Following AI trends
- Keeping an eye on competitors
- Continuous AI tool development
- Continuous team education
- Following relevant regulations and laws
We have highlighted the key components of AI transformation in detail. Apart from this, a successful process of AI Transformation requires more additional elements such as choosing relevant technology and tools, experienced engineers, and data privacy regulations. Here is a more extensive list of AI Transformation Components with a brief outline of their features.
AI Transformation Components | Steps to be implemented |
---|---|
1. Strategy and Vision |
|
2. Data Infrastructure |
|
3. Technology and Tools |
|
4. Talent and Skills |
|
5. Processes and Workflows |
|
6. Governance and Ethics |
|
7. Culture and Change Management |
|
8. Measurement and Improvement |
|
9. Use Cases and Applications |
|
Why are Companies Investing in AI?
Another question bothering business owners considering AI transformation is “Will investing in AI transformation pay off eventually?” Based on our experience, the integration of AI in digital transformation initiatives brings numerous benefits. AI offers potent tools that:
- Reduce cognitive load
- Improve system tracking and monitoring
- Provide simulation opportunities for better testing and QA
- Provide data-driven business insights
- Optimize operations
- Improve customer experiences
- Enable organizations to automate tasks
- Ensure more effective decision-making
All this can provide businesses with a competitive edge and certain internal and external benefits.
1. Improved Operational Efficiency
AI automates repetitive and routine tasks, speeding up processes and allowing human resources to concentrate on more complex activities. Moreover, AI-powered robotic process automation (RPA) tools, such as Automation Anywhere, Pega, or Tungsten Automation, offering multiple connectors and APIs, will facilitate the integration of innovations with your legacy systems, so that AI transformation feels more smooth and natural.
Find out more about RPA tools and how their adoption can change your business in our extensive blog piece on Robotic Process Automation.
Additionally, AI’s analytic capabilities can help reevaluate your existing workflows by identifying unseen patterns, spotting blockers, and suggesting more efficient approaches. AI algorithms are also useful in adjusting processes, schedules, and resource allocation to the demand and availability indicators.
2. Cost Savings and Resource Optimization
As a result of operational improvements, businesses can reduce their running expenses and start using available resources more wisely. ML-based demand forecasting and performance metrics tools provide plenty of data to be used for more accurate resource and effort allocation.
Also, AI investments pay off as automation tools help decrease labor costs. For example, natural language processing (NLP) technologies that power numerous document digitization and data extraction applications automate and speed up the processing and conversion of paper documentation into digital versions, thus reducing the need for hours of manual work. This way, business owners can either cut expenses or find costs that can be directed towards strategic initiatives like research and development or technological advancement instead of operational vortex.
3. Mitigation of Risks
For any business to thrive, it needs a development strategy that accounts for potential risks in addition to opportunities. AI-powered analytics and forecasting tools can be used to research and predict market and business trends, both promising and threatening. Being aware of uncertainties ahead is also a competitive advantage!
Another risk-mitigation application of AI tools in business is predictive maintenance of your systems, be it software, hardware, machinery, or fleet. These tools will track their performance, spot suspicious behavior patterns, and notify you of maintenance needs way ahead of a major breakdown. This way, you’ll ensure the functionality and longevity of your systems, as well as 100% business continuity, with minimal losses or expenses.
4. Personalized Customer Experiences
While most businesses are using customer-centric business models, AI delivers a comprehensive view of the customer. Relying on extensive customer data, AI-powered marketing, and sales tools recognize clients’ behavioral patterns and trends. This empowers business leaders to tailor their offerings, marketing strategies, and customer journeys to correspond to the individual preferences and expectations of their audiences. A multitude of companies are already benefiting from AI chatbots, self-service tools, and virtual assistants that yield increased lead generation, more personalized sales communications, and more efficient handling of customer inquiries.
5. Enhanced Decision Making
Discussing the benefits of AI transformation for a business, we can not skip the role of analytics in data-driven decision making. AI-powered analytics facilitate rapid and precise examination of extensive data sets, identifying trends, patterns, and anomalies, summarizing and visualizing data, explicating causes and effects, and forecasting the outcomes. Machine learning significantly contributes to improving analytics by:
- Automating the data analysis process
- Creating analytical models for extracting deeper insights
- Continuously enhancing the analytics workflow through adaptive learning capabilities.
As a result of such data processing, company managers and executives get a clear view of their position in the market, emerging opportunities and threats, internal bottlenecks, and working solutions, and so on, which provides enough awareness for making the right strategic decisions.
6. Competitive Advantage
Companies also decide to invest in AI transformation because AI technologies can help businesses outrun competitors in different industries and domains. Here are some examples of how AI can help you transform your processes, products, and services to stand out on the market:
- Marketing and Sales: AI can help you understand your customers better and deliver more relevant and engaging experiences. AI can help you segment your customers, predict their behavior and preferences, and tailor your messages and offers accordingly.
- Product Development and Innovation: using AI, you can experiment more to enhance your products and services with new features and functionalities. AI can help you design and test your products using generative design and simulation techniques.
- Operations and Supply Chain: improve your operations and workflows faster and more efficiently with AI. Use data generated by your business to optimize processes, resources, and inventory through predictive analytics and A-driven automation.
- Customer Service and Support: AI can help you provide better customer service and support with more convenience and satisfaction. AI can help you automate your customer interactions using AI tools such as chatbots and voice assistants.
Understanding the Technology behind AI Transformation
Artificial Intelligence is a broad field of research and development within computer science that covers a range of more narrow-focused disciplines, including machine learning, deep learning, data science, natural language processing, and others. Depending on the goal or task we want to complete, we can resort to different approaches and aspects of AI. Thus, to understand how AI can help a business in solving a specific problem, it’s good to know the technologies standing behind AI transformation solutions.
In the table below we list the key technologies used when implementing AI solutions and briefly explain what they are.
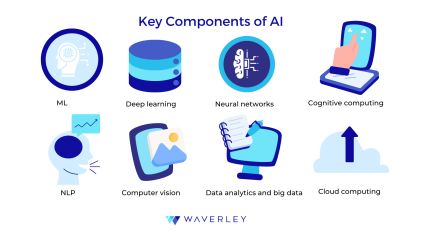
Technology | Description |
---|---|
Machine learning | ML is a subfield of AI that relies on algorithms that can train on data, producing computer models that learn and improve from previous sets of experiences to complete complex cognitive tasks, such as identification, classification, generalization, prediction, etc. |
Deep learning | DL is a subset of ML that learns to complete complex tasks with more accuracy than ML by processing large amounts of data with the help of artificial deep neural networks. |
Neural networks | Neural networks are computer systems that are loosely modeled on neural connections in the human brain and enable deep learning. |
Cognitive computing | Cognitive computing aims to recreate the human thought process in a computer model. It seeks to imitate and improve the interaction between humans and machines by understanding human language and the meaning of images. |
Natural language processing | NLP is a toolset that allows computers to comprehend, recognize, interpret, and produce human language and speech. The most widely used example of such tools today is GPT – a set of large language models for text processing and generation. |
Computer vision | Computer vision employs deep learning and pattern identification to interpret image content (graphs, tables, PDF pictures, and videos). |
Data analytics and big data | Data analytics aims to structure historical data and use it to find patterns, draw conclusions, and predict future trends. ML algorithms rely on the results of data analytics for training and creating computer models that can process new unstructured data to deliver insights, detect anomalies, produce advanced visualizations, provide automation, and make decisions. |
Cloud computing | One of the key benefits of cloud computing in the context of AI is scalability. Cloud platforms offer virtually unlimited computational resources and data storage, allowing organizations to scale AI applications up or down based on demand. |
9 Steps to Implement AI – Checklist for AI Transformation
Now that we’ve covered the benefits of implementing AI in business and the main technologies backing this process, we can get down to action. The Artificial intelligence transformation of your business is a gradual process that requires preparation and following certain steps to achieve maximum efficiency. But don’t get overwhelmed – in this section we will guide you through each step of this journey, highlighting the important aspects.
At the same time, it’s worth mentioning that each use case and solution is unique, and such would be the specific recommendations for a particular project. Therefore, here we provide the general advice for the successful AI implementation.
Based on our AI solution development experience as well as some best practices described by AI technology experts in the AI Transformation Playbook, we suggest the optimal action plan for a successful AI implementation. Now, let’s look at the process of digital transformation with AI step by step.
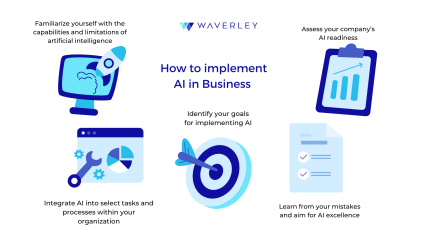
1. Objectives Definition
- We recommend starting with understanding the objectives of your business. These could include improving customer service, increasing efficiency, reducing costs, or entering new markets.
- After this is set, specify what you aim to achieve with AI. For example, improving customer experience, reducing costs, automating routine tasks, etc. During this stage, establish clear, measurable targets for each AI initiative. These could include metrics such as cost savings, revenue growth, customer satisfaction scores, or productivity gains.
2. Technology Assessment
- You can begin by cataloging existing technologies, systems, and software used within the organization. Also, explore potential AI applications and use cases across different business functions, such as customer service, marketing, operations, and finance.
- Assess the organization’s readiness to adopt AI technologies, including factors such as data availability, infrastructure, talent, and cultural acceptance.
3. Data Readiness and Preparation
- Data preparation starts with identifying the specific data needed to support AI initiatives based on the identified use cases and objectives. This might include structured data from databases, unstructured data from text documents or images, or streaming data from IoT devices.
- After one might want to evaluate the quality of the data, it’s also important to use data-cleaning techniques if it’s needed. This step is critical for ensuring the accuracy and reliability of AI models.
- Establish data governance policies and procedures to ensure the security, privacy, and compliance of data used for AI applications.
4. AI Technology Selection
- Evaluate the technical requirements for each AI use case, including the type and volume of data needed, the ML algorithms or models required to complete the task, and the computational resources necessary to support the solution.
- Research and explore the various AI technologies and tools available in the market that are relevant to your use cases. This may include machine learning frameworks (e.g., TensorFlow, PyTorch), pre-trained models (e.g., BERT, ResNet), or AI platforms (e.g., Azure AI, Google Cloud AI).
- Evaluate vendor solutions that offer AI technologies or platforms tailored to your specific use cases. Consider factors such as functionality, scalability, ease of integration, vendor reputation, customer support, and pricing.
5. Build or Acquire AI Models
- It’s advised to assess your in-house expertise. It means evaluating your organization’s internal expertise in AI, including data science, machine learning, and software development capabilities.
- Determine if you need or have the necessary skills and resources to build AI models from scratch.
- You can also explore external resources, such as AI vendors, open-source projects, or consulting firms specializing in AI development.
6. Pilot Development, Testing, and Validation
- It makes sense to identify participants who will be involved in the pilot development and testing phase, first. This may include end-users, subject matter experts, IT professionals, and project managers responsible for overseeing the pilot implementation.
- Execute the pilot testing phase and gather feedback from pilot participants, monitor system performance, and collect relevant data to evaluate the effectiveness and usability of the AI solution.
- Based on the feedback and insights gathered during pilot testing, iterate and refine the AI solution as needed.
7. Solution Development and Scaling
- Select scalable technologies and platforms for building and deploying your AI solution.
- Consider architectural patterns, design principles, and best practices for building scalable systems, such as microservices architecture, distributed computing, and horizontal scaling.
- Choose tools, frameworks, and infrastructure that can easily scale to accommodate increased demand without sacrificing performance or reliability.
8. Data Privacy and Security Considerations
- Data Privacy Impact Assessments (DPIAs) are advised to evaluate the privacy risks associated with AI projects.
- Encrypt sensitive data at rest and in transit to protect it from unauthorized access and interception.
- Stay updated on relevant data privacy and security regulations, such as GDPR (General Regulation on the Protection of Personal Data) as well as your local guidelines and legislation in force regarding the use of AI technologies.
9. Integration with Existing Systems
- Identify integration points where AI technologies will interact with existing systems.
- Determine whether data ingestion, preprocessing, inference, or post-processing is required, and identify the APIs or interfaces needed for integration.
- Integrate AI models with existing systems through APIs or web services.
Emerging Trends in AI Strategy
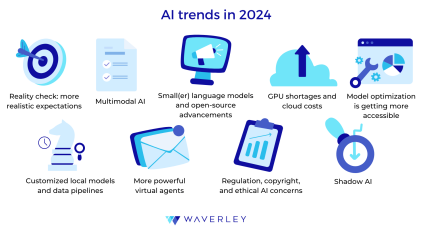
Why is it important to stay in the loop regarding AI and its trends? IBM experts outlined that 2022 was the year that generative AI exploded into public knowledge, and starting from 2023 it began to take root in the business world. Consequently, 2024 will be the year of AI, as researchers and enterprises seek to establish how this evolutionary technological leap can be most practically integrated into our everyday lives.
Experts predict the growth of multimodal AI models and shadow AI usage. On the other hand, ethical AI concerns will become more urgent in 2024, as finding solutions regarding this issue will be at the forefront. Let’s have a look at general AI trends in the following year.
- At the time when generative AI became available to the public, a typical business leader’s knowledge came mostly from marketing or news. The experience of adopting generative AI was limited to using chatGPT or offering chatGPT integration services, but now the business community has a more refined understanding of AI-powered solutions.
- Interestingly, Deloitte’s ‘State of Generated AI in the Enterprise’ report from Q1 2024 indicated that many leaders ‘expect substantial transformative impacts in the short term’.
- According to a recent IBM survey of over 1,000 employees at enterprise-scale companies, the top three factors driving AI adoption were advances in AI tools that make them more accessible, the need to reduce costs and automate key processes, and the increasing amount of AI embedded into standard off-the-shelf business applications.
- Enhancing performance for multi-models. Models like OpenAI’s GPT-4V or Google’s Gemini, as well as open-source models like LLaVa, Adept, or Qwen-VL, can move freely between natural language processing and computer vision tasks.
- Evidently, the power of open models will continue to grow. For instance, in December of 2023, Mistral released ‘Mixtral’ a mixture of expert (MoE) models integrating 8 neural networks, each with 7 billion parameters. The advances in smaller models will have certain benefits:
- Making AI more explainable
- Making AI more widely available
- Allowing AI to run locally on smaller devices
Importantly, company and business leaders should be aware of ‘shadow AI’ — basically, the implementation of generative AI in the workplace without going through IT for approval or oversight.
Are there any factors that stop or slow down digital transformation with AI? Frankly speaking, the ambiguity in the regulatory environment slows down the AI transformation processes. Starting from 2023 we have begun to make laws and regulations. In December 2023, the European Union (EU) reached a temporary agreement on the Artificial Intelligence Act. It regulates the creation and usage of facial recognition databases, biometric categorization systems with the potential for discriminatory bias, or ‘social scoring’ systems, and the use of AI for social or economic manipulation. It also seeks to define a category of “high-risk” AI systems, with the potential to threaten safety and fundamental rights.
Now let’s have a deeper look at the latest developments shaping AI strategy. These trends will shape the way companies and organizations adapt to the new and changing business landscape.
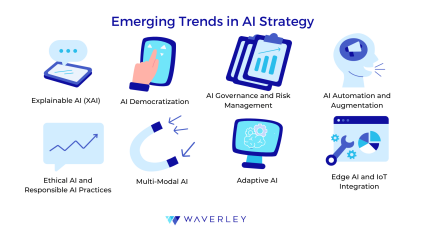
Trends | Explanation |
---|---|
Explainable AI (XAI) | Explainable AI is gaining traction as organizations seek to understand and interpret the decisions made by AI systems. XAI techniques aim to make AI models more transparent and interpretable, enabling stakeholders to trust AI-driven insights and understand the reasoning behind AI recommendations. |
AI Democratization | The democratization of AI is making AI technologies more accessible to organizations of all sizes and industries. Cloud providers and AI platforms are offering pre-built AI models, tools, and services that enable organizations to easily integrate AI into their existing workflows without requiring extensive technical expertise. |
AI Governance and Risk Management | With the increasing reliance on AI, organizations are paying more attention to AI governance and risk management. This includes establishing policies, procedures, and controls to ensure compliance with regulations, mitigate risks associated with AI deployment, and manage ethical considerations. |
AI Automation and Augmentation | AI is being increasingly used for automation and augmentation of human capabilities across various industries. Organizations are leveraging AI-powered automation to streamline processes, improve efficiency, and reduce operational costs. Additionally, AI augmentation is enhancing human capabilities by providing insights, recommendations, and decision support. |
Ethical AI and Responsible AI Practices | There is growing recognition of the importance of ethical considerations in AI development and deployment. Organizations are increasingly adopting responsible AI practices to ensure fairness, transparency, accountability, and privacy in AI systems. Regulatory bodies are also starting to introduce guidelines and regulations to govern AI usage. |
Edge AI and IoT Integration | Edge AI, which involves running AI algorithms on edge devices, is becoming more prevalent as organizations seek to process data closer to its source, achieving real-time response. This is particularly relevant in IoT (Internet of Things) applications, where AI-powered edge devices can analyze sensor data, detect anomalies, objects, etc., and trigger actions autonomously. |
Multi-Modal AI | Multi-modal AI, which involves processing and analyzing data from multiple sources such as text, images, and audio, is becoming increasingly important for solving complex problems and extracting more meaningful insights. Organizations are exploring ways to integrate multi-modal AI into their applications to enhance user experiences and improve decision-making. |
Adaptive AI | Adaptive AI techniques are enabling AI systems to learn and evolve in response to changing environments and new data. This allows organizations to build more robust and resilient AI systems that can adapt to evolving business needs and unforeseen challenges. |
Conclusion
As you move forward on your AI transformation journey, it’s important to remember that success is not defined by the complexity of the AI algorithms you deploy, but by the value they deliver to your business and your customers. Keep the focus on solving real-world problems, driving innovation, and creating tangible business outcomes.
To achieve this, choosing the right AI integration strategy is crucial. Another key point is to collaborate with experts in AI digital transformation. Getting our consulting services can help you discover the transformative power of artificial intelligence.
FAQ
How to use AI in digital transformation?
Here are the ways how to use AI in digital transformation: predictive and data analytics, personalization of customer experiences, automation processes, NLP technologies, computer vision technologies, fraud detection, etc.
What is AI transformation, and why is it important for businesses?
The AI transformation process includes the integration of AI technologies into different aspects of a business. It’s important for businesses to drive innovation, improve the decision-making process, and reduce costs.
What are the key components of a successful AI transformation strategy?
A successful strategy for digital transformation with AI involves careful planning, execution, and integration. Business owners must set clear business goals, develop a comprehensive data strategy, build a skilled team, and invest in the technology infrastructure to ensure the successful implementation of artificial intelligence transformation.
How can AI help businesses enhance decision-making?
AI can help businesses enhance decision-making in multiple ways, such as data analysis and predictive analytics, automation of routine tasks, real-time insights and notifications, scenario planning, creating personalized customer experiences, etc.
How can businesses measure the success of their AI implementation?
To measure the success of the AI transformation process businesses can use different metrics such as performance metrics, business impact metrics, ROI and cost-benefit analysis, as well as customer feedback collection.
How can businesses stay informed about emerging AI trends to continuously adapt their strategies?
There are various means for businesses to stay informed about emerging AI trends for example, visiting industry conferences and events, following online resources and publications related to your industry, studying academic research and journals, and establishing partnerships and collaborations with technology vendors and startups. By leveraging these approaches, businesses can stay informed about emerging AI trends.
Start your AI transformation journey